Introduction
In the Python world Matplotlib has become the de-facto standard for drawing charts. While getting started is easy, as developers can create charts with only a few lines of code, Matplotlib can be a little quirky sometimes. In this article we would like to demonstrate that it is actually not so difficult to integrate Matplotlib charts in a Qt application written in PySide. It is even possible to customize the chart by tweaking the default behavior, exchange menu entries or add specific paint logic.
If you are more interested in a general overview of using Qt (and Qt Creator) together with Python or if you need some arguments to start, you may want to look also into the blog post written by my collegaue Kai Uwe Broulik. Now let’s start with an example in the next section.
A first example
To run the examples, just create a virtual environment and install the dependencies PySide6, Matplotlib and Pandas.
python -m venv env
source env/bin/activate
pip install pyside6 matplotlib pandas
basysKom is a company that is enthuasiastic about Open Source and Linux – so using a dataset to classify penguins seems to be a natural fit for this example: You can download it from Github. Each row in the dataset belongs to a penguin of one of the species adelie, gentoo (yeah, the eponymous penguin species of the Linux distribution) and chinstrap. The columns store measurements of bill length and thickness, flipper length and the body mass next to some other features like island habitat and year of observation. If you want to know more about the data, take a look at the official page.
Following is the complete code to load the dataset, remove rows with missing values and visualize the features with a scatter plot. The feature on the x- and y-axis can be selected by choosing the corresponding entry in the QComboBox.
import sys
import pandas as pd
from PySide6.QtWidgets import (
QApplication, QMenu, QWidget, QVBoxLayout,
QSizePolicy, QComboBox, QLabel, QPushButton
)
from PySide6.QtGui import QAction, QPainter, QColor
from PySide6.QtCore import Qt, QObject, QRectF
import matplotlib.pyplot as plt
from matplotlib.backends.backend_qtagg import NavigationToolbar2QT as NavigationToolbar
from matplotlib.backends.backend_qtagg import FigureCanvas
from matplotlib.figure import Figure
class DataVisWidget(FigureCanvas):
def __init__(self, parent=None, width=300, height=300, dpi=100):
self.fig = Figure(figsize=(width / dpi, height / dpi))
super().__init__(self.fig)
self.ax = self.fig.subplots()
if parent is not None:
self.setParent(parent)
def update_plot(self, df, column_name_x, column_name_y, column_name_label):
categories = df[column_name_label].astype("category")
codes = categories.cat.codes
labels = categories.cat.categories
cmap = plt.get_cmap("Set2")
colormap = [cmap(code) for code in codes]
self.ax.clear()
self.ax.scatter(df[column_name_x], df[column_name_y], c=colormap)
self.ax.legend(handles=[
plt.Line2D([], [], marker='o', linestyle='', color=cmap(i), label=str(label))
for i, label in enumerate(labels)
])
self.ax.set_xlabel(column_name_x)
self.ax.set_ylabel(column_name_y)
self.request_plot_update()
def request_plot_update(self):
self.fig.canvas.draw()
self.update()
def main():
# load palmer penguin dataset
df = pd.read_csv("./data/penguins.csv")
columns = {
"bill_length_mm": "Bill Length (mm)",
"bill_depth_mm": "Bill Depth (mm)",
"flipper_length_mm": "Flipper Length (mm)",
"body_mass_g": "Body Mass"
}
target_column = "species"
subset = list(columns.keys()) + [target_column]
#remove rows with zero values
df.dropna(subset=subset, inplace=True)
app = QApplication(sys.argv)
app.setApplicationDisplayName(QObject.tr("Palmer Penguins"))
main_widget = QWidget()
layout = QVBoxLayout(main_widget)
vis_widget = DataVisWidget(main_widget, width=800, height=600)
vis_widget.setSizePolicy(QSizePolicy.Policy.Expanding, QSizePolicy.Policy.Expanding)
toolbar = NavigationToolbar(vis_widget, main_widget)
combobox_x = QComboBox(main_widget)
combobox_y = QComboBox(main_widget)
for feature, name in columns.items():
combobox_x.addItem(QObject.tr(name), feature)
combobox_y.addItem(QObject.tr(name), feature)
def update_plot():
vis_widget.update_plot(df, combobox_x.currentData(), combobox_y.currentData(), target_column)
combobox_x.currentIndexChanged.connect(update_plot)
combobox_y.currentIndexChanged.connect(update_plot)
combobox_y.setCurrentIndex(1)
button = QPushButton(QObject.tr("Quit"), main_widget)
button.clicked.connect(app.quit)
layout.addWidget(toolbar)
layout.addWidget(QLabel(QObject.tr("X Axis:")))
layout.addWidget(combobox_x)
layout.addWidget(QLabel(QObject.tr("Y Axis:")))
layout.addWidget(combobox_y)
layout.addWidget(vis_widget)
layout.addWidget(button)
main_widget.show()
app.exec()
if __name__ == "__main__":
main()
After starting the application, you should see something similar to the screenshot:
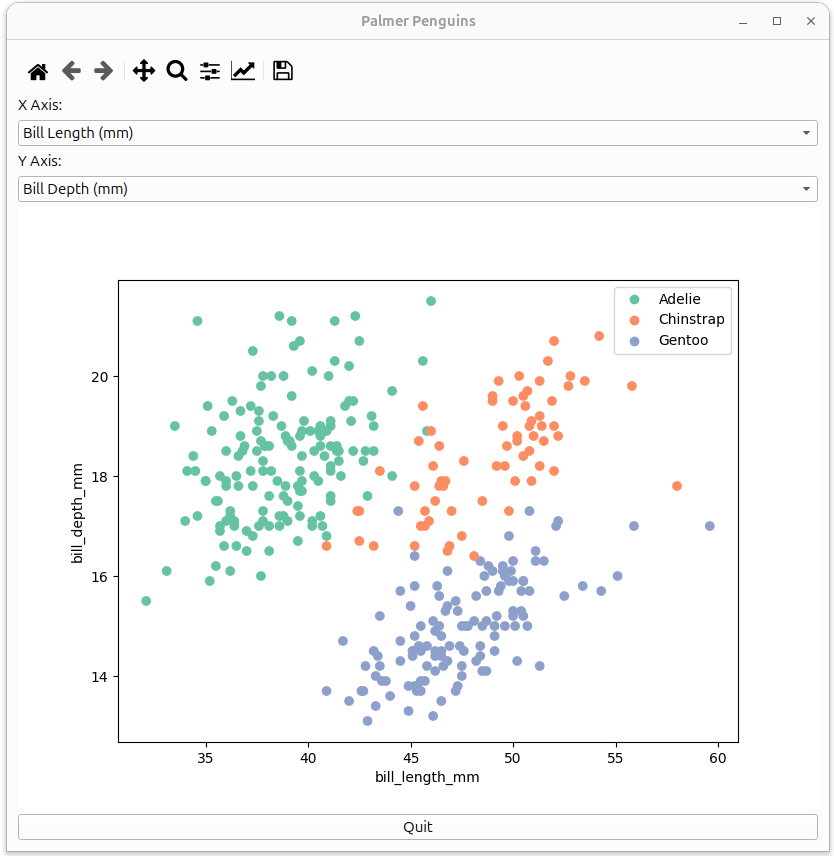
So how does the integration wit Qt/PySide6 work? Matplotlib offers different render backends of which the QtAgg backend is used by default. Extend the rendering canvas as follows: Import FigureCanvas from matplotlib.backends.backend_qtagg and let your class inherit from it, then create a Figure object in the constructor. When adding one or more subplots to the figure, store the returned axes object (or list of axes objects, if you are creating multiple subplots) as a class member. Use the axes object for configuring and rendering the plot as it is done in the update_plot() method. As a FigureCanvas based class inherits also from QWidget, it can be integrated into a Qt application like any other QWidget.
Adding Matplotlib’s NavigationToolbar2QT makes the plot interactive: In the snippet above, we just instantiate the toolbar, pass the FigureCanvas based instance as a parameter and add everything to our layout. That’s it. Now, the user is able to pan and zoom the plot and save it as a png image.
Unfortunately it is not straightforward to deviate from this standard appearance: The rubberband used to select the zoom region is hardcoded, the toolbar is ready-made and doesn’t offer many options to tweak its behavior. So how it is possible to add a bit more customization on top?
Customizing Matplotlibs
As FigureCanvas is QWidget based, you can override all the well-known event handlers like paintEvent(), contextMenuEvent(), etc. … Additionally, Matplotlib offers its own callbacks for event handling.
self.fig.canvas.mpl_connect("button_press_event", self.on_press)
self.fig.canvas.mpl_connect("motion_notify_event", self.on_notify)
self.fig.canvas.mpl_connect("button_release_event", self.on_release)
So, what is the advantage to use these specialized Matplotlib event handlers over Qt’s own mousePressEvent(), mouseReleaseEvent(), etc.? Matplotlib passes an event parameter to the callback handlers that stores mouse coordinates in both widget space (mouse position inside the widget) and plot space (mouse position relative to the x- and y-axes, i.e. translated to the space of the plotted function) along with useful information such as whether the mouse is inside or outside the chart’s axes.
This knowledge makes it easy to implement our own rubberband logic. Here you can find the updated DataVisWidget (everything else stays the same):
class DataVisWidget(FigureCanvas):
def __init__(self, parent=None, width=300, height=300, dpi=100):
self.fig = Figure(figsize=(width / dpi, height / dpi))
super().__init__(self.fig)
self.ax = self.fig.subplots()
if parent is not None:
self.setParent(parent)
self.fig.canvas.mpl_connect("button_press_event", self.on_press)
self.fig.canvas.mpl_connect("motion_notify_event", self.on_notify)
self.fig.canvas.mpl_connect("button_release_event", self.on_release)
self.reset_plot_action = QAction("Reset Plot", self)
self.reset_plot_action.triggered.connect(self.reset_plot)
self.setContextMenuPolicy(Qt.ContextMenuPolicy.ActionsContextMenu)
self.addAction(self.reset_plot_action)
self.rubberband_rect = None
self.data_rect = None
self.x_lim = None
self.y_lim = None
def request_plot_update(self):
self.fig.canvas.draw()
self.update()
def update_plot(self, df, column_name_x, column_name_y, column_name_label):
categories = df[column_name_label].astype("category")
codes = categories.cat.codes
labels = categories.cat.categories
cmap = plt.get_cmap("Set2")
colormap = [cmap(code) for code in codes]
self.ax.clear()
self.ax.scatter(df[column_name_x], df[column_name_y], c=colormap)
self.ax.legend(handles=[
plt.Line2D([], [], marker='o', linestyle='', color=cmap(i), label=str(label))
for i, label in enumerate(labels)
])
self.ax.set_xlabel(column_name_x)
self.ax.set_ylabel(column_name_y)
self.x_lim = self.ax.get_xlim()
self.y_lim = self.ax.get_ylim()
self.request_plot_update()
def on_press(self, event):
if self.rubberband_rect is not None or self.data_rect is not None:
return
if event.button != 1: # left button
return
self.rubberband_rect = [event.x, event.y, event.x, event.y]
self.data_rect = [event.xdata, event.ydata, event.xdata, event.ydata]
self.update()
def on_notify(self, event):
if self.rubberband_rect is None or self.data_rect is None:
return
if event.inaxes:
self.rubberband_rect[2] = event.x
self.rubberband_rect[3] = event.y
self.data_rect[2] = event.xdata
self.data_rect[3] = event.ydata
self.update()
def on_release(self, event):
if self.data_rect is not None:
x1, y1, x2, y2 = self.data_rect
if x1 != x2 and y1 != y2:
self.ax.set_xlim(sorted([x1, x2]))
self.ax.set_ylim(sorted([y1, y2]))
self.rubberband_rect = None
self.data_rect = None
self.fig.canvas.draw()
self.update()
def reset_plot(self):
if self.x_lim is None or self.y_lim is None:
return
self.ax.set_xlim(self.x_lim)
self.ax.set_ylim(self.y_lim)
self.request_plot_update()
def paintEvent(self, event):
super().paintEvent(event)
if self.rubberband_rect is not None:
p = QPainter(self)
p.setBrush(QColor(0, 0, 127, 64))
x1, y1, x2, y2 = self.rubberband_rect
box = QRectF()
box.setCoords(x1, self.height() - y1, x2, self.height() - y2)
p.drawRect(box)
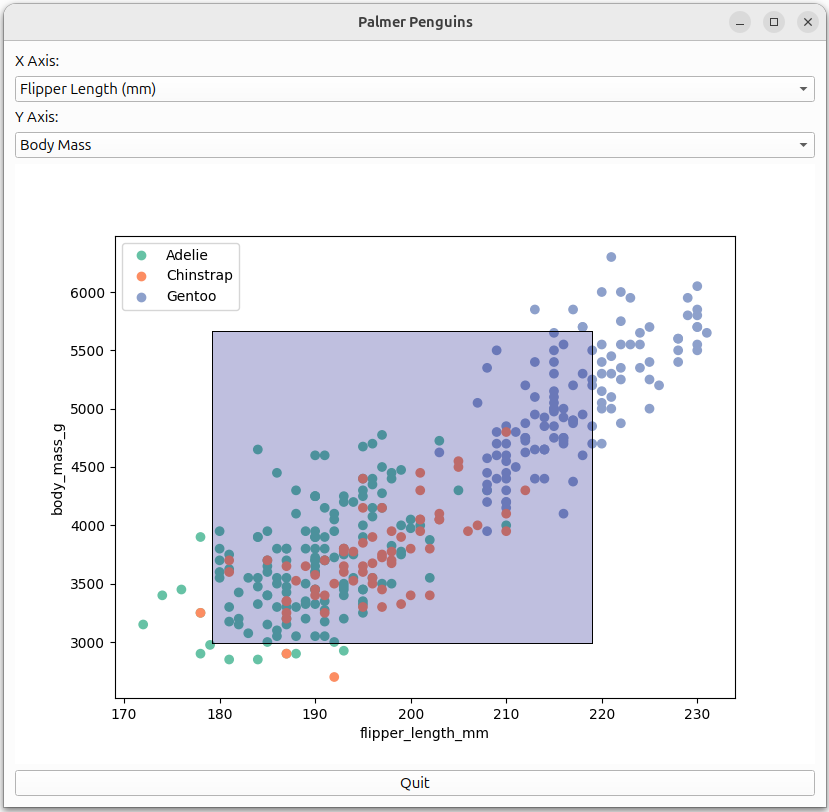
Other plotting libararies
# Don't forget to install Seaborn first: pip install seaborn
import seaborn as sns
# The rest of the code remains the same
# ...
# The updated method using Seaborn
def update_plot(self, df, column_name_x, column_name_y, column_name_label):
self.ax.clear()
sns.scatterplot(data=df, x = column_name_x, y = column_name_y, hue=column_name_label, ax=self.ax)
self.x_lim = self.ax.get_xlim()
self.y_lim = self.ax.get_ylim()
self.request_plot_update()
Note the ax parameter that we have passed to Seaborn’s scatterplot() method: If ax is not None, Seaborn uses the given axis to draw into. This is important. Without the ax parameter, Seaborn tries to create a new axis and therefore a new chart.
Matplotlib and Seaborn are among the most popular, but certainly far from being the only plotting libraries in the Python world. There exists others like Ploty and Bokeh, that render interactive plots by default. But they output HTML and JavaScript, meaning that a renderer for web content like QWebEngine is needed to embed them into a PySide6 application.